ChatGPT awes people with remarkably detailed, well-articulated responses to diverse questions spanning topics from biology to history to pop culture trivia.
This ability to synthesize coherent answers is powered by ingesting tremendous knowledge during pretraining – over a hundred billion words from websites, books, and more.
In this post, we’ll unpack how exposure to massive datasets enables knowledge synthesis along with ChatGPT’s reasoning capacities.
Pretraining Objective: Knowledge Ingestion
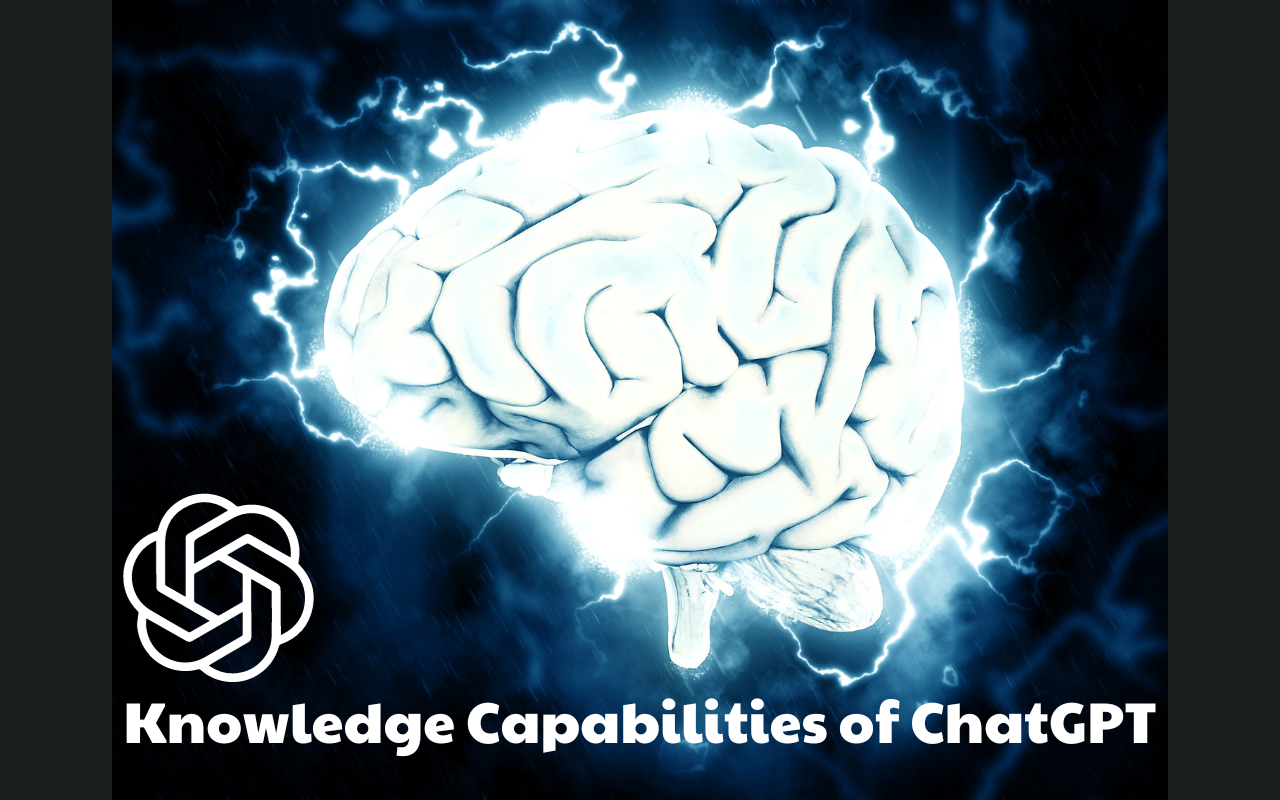
ChatGPT owes its broad knowledge base to the sheer scale of text consumed during pretraining – Orders of magnitude more data than previous language models.
This exposes the model to a vast array of topics through:
- Extracting key details and relationships from billions of web pages
- Ingesting full text from over 50,000 books spanning genres
- Analyzing intricacies in diverse articles and written mediums
The resulting breadth allows ChatGPT to synthesize responses drawing from an exceptionally wide knowledge base.
Even niche topics often have relevant examples within their training data to extrapolate from.
Architectural Adaptations for Reasoning
Of course, simply retrieving factual nuggets isn’t sufficient – true reasoning requires contextual application rather than randomly listing tidbits.
That’s why alongside scale, architectural innovations equip ChatGPT to logically synthesize coherent, meaningful responses:
- Causal language modeling objectives foster an understanding of causality
- Self-consistency training avoids blatant contradictions
- Memory augmentations track earlier dialogue for consistency
Combined, these mechanisms ground ChatGPT’s knowledge in logic – allowing sensibly formulated answers even about unfamiliar topics.
The model displays actual reasoning capacities rather than just language prediction.
Ongoing Knowledge Expansion
Looking ahead, OpenAI continues rapidly expanding ChatGPT’s knowledge through ongoing pretraining on new data.
Recent additions cover topics like COVID-19, Web3, AI safety research, and more – helping keep responses updated.
The ability to efficiently ingest emerging information illustrates the scale advantages of foundation models. ChatGPT assimilates new data better than narrower AI.
As dataset breadth crosses a trillion words, expect knowledge capabilities to reach new heights. However, avoiding overconfidence in responses remains an ongoing challenge.
However responsibly applied, expansive knowledge synthesis could enable ChatGPT to assist people in tremendously beneficial ways. The potential remains vast.